Bridge Health Modeling
Bridges are the national critical infrastructures. However, according to ASCE bridge report card in 2009, more than 26% of the nation’s bridges are either functionally obsolete or structurally deficient. Current bridge management predominantly relies on visual inspection, which cannot uncover the hidden deteriorations. Bridge Health Monitoring and Modeling (BHM) is the innovative approach to assess the bridge health performance. Most of BHM programs are limited to data collection. In this project, Bentley Systems and STRAAM Corporation have jointly conducted the pilot study on the bridge health performance for the approach span of Verrazano Narrows Bridge in New York City. With the detailed bridge design drawings, the finite element model (FEM) was built by using Bentley STAAD Pro. The constructed FEM was employed to optimize the sensor placement of the bridge span. The optimized sensor location helped to determine and finalize the placement of the accelerometers, which were placed on the bridge to record the dynamic responses of the bridge span under normal traffic condition during the day. The recorded responses were processed to extract the primary modes and the frequencies that were used for FEM calibration, which was dramatically facilitated by the optimization runs conducted on a High Performance Computing (HPC) cluster using Darwin Optimization Framework. The calibrated FE model can serve as a valuable knowledge tool for the bridge condition assessment and the solution evaluation of prognostic bridge maintenance.
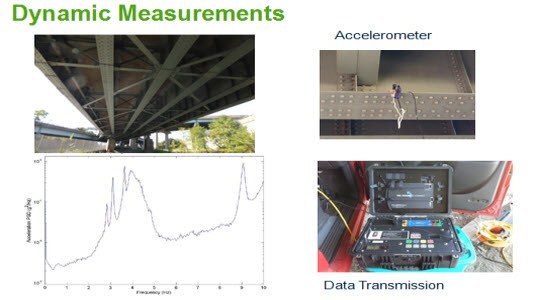
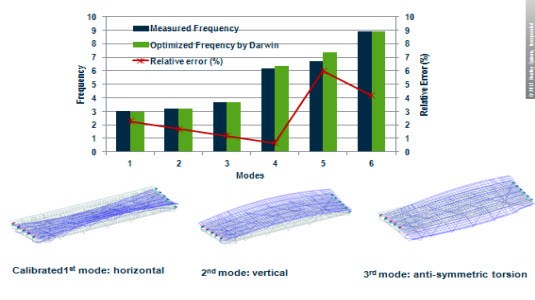
Published Papers:
-
Wu, Z. Y., Yi, X., Zhao, J., Jeary, A., Winant, T., Love, R. and Bhide, S. (2013). “Optimizing Sensor Placement and Dynamic Measurements of Verrazano Narrows Bridge Span.” SHMII-6, December 9-11, 2013, Hong Kong, China.
-
Wu, Z. Y., Zhao, Love, R., Bhide, S. J., Jeary, A. and Winant, T. (2013). "Optimized Calibration of Finite Element Model for Verrazano Narrows Bridge Span", SHMII-6, December 9-11, 2013, Hong Kong, China.
Signal Processing for Modal Parameter Identification
Dynamic responses of civil structures under ambient environment and external loading condition, e.g. earthquake, contain the important information of the structural health condition. The responses are usually measured (in short term) or monitored (in long term) by using accelerometers, which record a large amount of raw data of accelerations. The data needs to be processed so that useful information, including natural frequencies and modal shapes, can be extracted for structural health evaluation and modeling. This project developed the algorithms for processing the data to identify dynamic signatures (natural frequency, damping ratio and mode shape) of a civil structure. The developed methods are applied to the UCLA Factor Building, a benchmark of building health monitoring, under both conditions of the ambient vibration and the earthquake vibration. The results obtained are compared with those previously published in literature. It is verified that the data processing framework we developed is effective and generic at modal parameter identification. It serves as a tool for extracting modal information from raw dynamic data for civil structures.
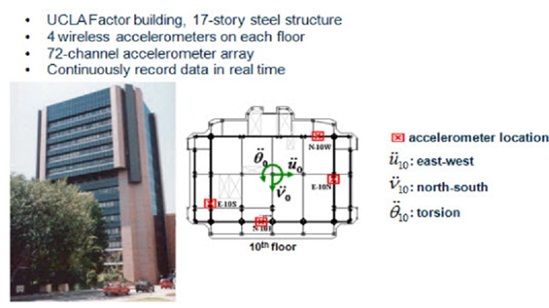
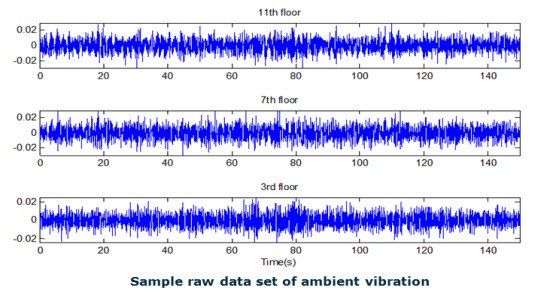
Structural Damage Detection
America’s infrastructure overall deteriorates to the low level with GPA of D. For instance, over 500,000 highway bridges with a span length greater than 25 feet, more than 26% of the bridges are either structurally deficient or functionally obsolete. Therefore, timely detecting its defect and the accumulated damage is essential for infrastructure owners and engineers to take preventive or proactive maintenance in order to avoid disaster like the bridge collapse of I-35 on Mississippi river in 2007, and also ensure that an infrastructure system is able to perform its current and future service as desired. This is usually achieved by undertaking Structure Health Monitoring (SHM). Over last decades, three types of damage detection methods have been investigated for SHM practice, namely physics-base methods, data-driven methods and statistics-based method. Physics-based method is to infer the physical characteristics of a structure system by solving an inverse problem, which correlates the corresponding mathematic model, e.g. finite element model, with the monitored responses. With FE model having been well-developed and widely-used by structural engineers, it is highly desirable to have an effective method for damage detection based on FE model. A new method has been developed by calibrating FE model. Possible structure damage is represented with a set of meaningful geometric parameters, the damage detection method is to search for the damaged elements and the corresponding material attributes so that the differences between the modeled and the measured static and modal responses is minimized. The process also helps engineers to identify a sound FE model for condition assessment of a structure system.
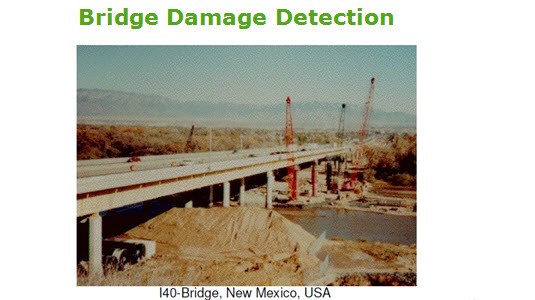
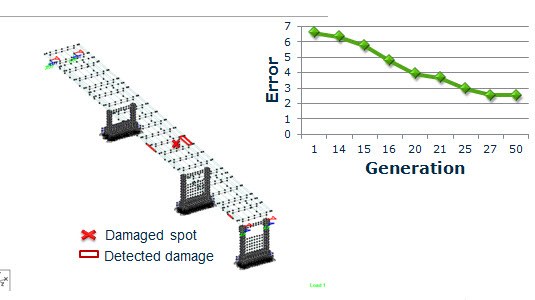
Published Papers:
- Wu, Z. Y., Xu, G., Mi, T. and Zhao, J. (2012) "High performance computing for damage detection of civil infrastructural systems", IABMAS-6, July 2012, Stresa, Italy.
-
Wu. Z. Y. & Xu G. (2011). "Effective Method for Locating Damage Elements by Parallel Optimization of Model Updating" SHMII-5, Cancun, Mexico.
Finite Element Model Calibration
Finite element (FE) model calibration is an essential task for infrastructural health modeling. A calibrated FE model can adequately represent the conditions of existing structures and yield reliable responses under the prescribed dynamic loadings. It is an important tool for engineers to assess the structural conditions and enable condition-based prognostic maintenance. However, FE model parameters such as material properties and geometric attributes for the built infrastructure are always subject to variations due to material imperfection, manufacturing/construction tolerance, deterioration over time and ambient effects, such as corrosion, temperature and humidity changes. Model identification by updating or calibrating the initial FE model with the field data, e.g. natural frequency and mode shapes extracted from the dynamic measurements, is a challenging task due to the large number of elements and uncertain boundary conditions. A FE model calibration method has been developed by employing the optimization technology to facilitate the calibration process. Using the static and dynamic measurements, the FE model calibration is defined to search for a set of the chosen structural parameters so that the difference between the FE model-analyzed and field-observed responses (natural frequencies and mode shapes) is minimized. It is an implicit nonlinear optimization problem and solved by using Darwin parallel optimization framework. The integrated FE model calibration tool has been successfully applied to a number of real structure cases.
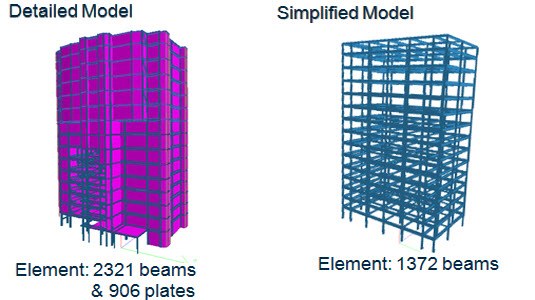
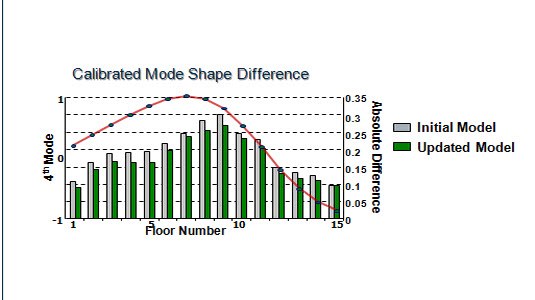
Published Papers:
-
Wu, Z. Y. and Xu, G. (2011). "Integrated Evolutionary Optimization Framework for Finite Element Model Identification" Proceedings of SMAR 2011, the 1st Middle East Conference on Smart Monitoring, Assessment and Rehabilitation of Civil Structure, Feb. 8-10, 2011, Dubai, UA
-
Zhao, J. and Wu, Z. Y. (2013) “Finite Element Model Calibration of UCLA Factor Building by Darwin Parallel Optimization.” SHMII-6, December 9-11, 2013, Hong Kong, China
Sensor Placement for Structural Health Monitoring
Structural health monitoring (SHM) is becoming an increasingly important approach for providing good data to come up with technically-sound solutions for life-cycle infrastructure management. SHM can be undertaken for a few hours, so-called short-term testing or measurement and continuously so-called long-term monitoring. Various sensors, wired and wireless, are used for measuring different parameters, such as acceleration, strain, displacement, deflection, corrosion, temperature and humidity etc., which are related to structural health performance. Among all the parameters, acceleration is the most important factor to be used for assessing the integrity of dynamic behavior of the bridge structure (and many other civil structures too). However, determining how many accelerometers are to be placed and where to place them is not straight forward, it usually relies on the engineering judgment. In this project, a sensor placement method is presented and applied to optimize the accelerometer placement. The sensor placement method is formulated to search for the sensor locations, represented as nodes in finite element model, to maximize the coverage of the dynamic integrity, which is defined as percentage of the detectable damage scenarios. Using Mont Carlo method and the finite element model, thousands of damage scenarios are automatically generated and analyzed, the calculated responses are used to form a sensitivity matrix, which is used to evaluate the sensor placement location. For a given number of sensors, the placement solution is optimized by using Darwin optimization framework a generic parallel optimization tool.
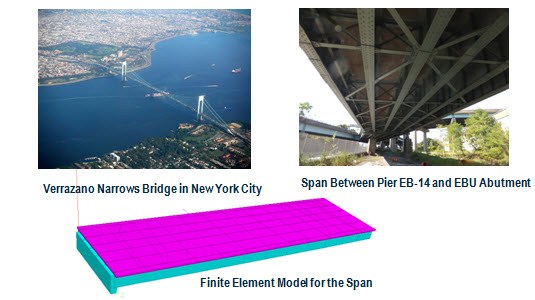
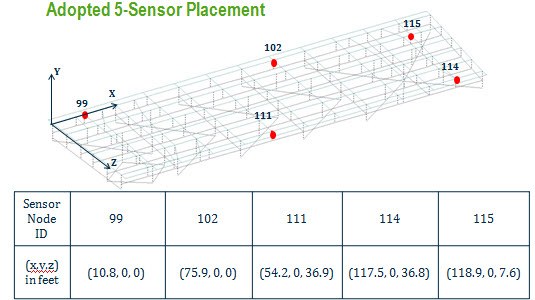
Published Papers:
- Wu, Z. Y., Yi, X., Zhao, J., Jeary, A., Winant, T., Love, R. and Bhide, S. (2013). “Optimizing Sensor Placement and Dynamic Measurements of Verrazano Narrows Bridge Span.” SHMII-6, December 9-11, 2013, Hong Kong, China.
Piezoelectric-based Method for Damage Identification
Condition assessment of bridges is necessary to ensure their safe operations. Although in USA bridges are normally inspected biennially, online structural damage detection or structural health monitoring systems are urgently needed. Due to the unique characteristics of piezoelectric transducers, they are employed in our project to develop a robust damage detection scheme. In this report, the vibration based damage detection method using modal frequencies is formulated to identify both the location and the severity of the damage in a benchmark structure emulating bridge component. Using the difference between the monitored modal frequencies and calculated baseline modal frequencies as input, first order and second order inverse identification algorithms are formulated to directly identify the change of the structural element properties such as Young’s modulus and cross-sectional area. A benchmark truss structure system is established to test the formulated identification algorithms. Furthermore, the statistical approaches are introduced to improve the robustness of identification algorithm in practical engineering structures and systems, where there exist uncertainties, such as the errors in the baseline finite element model and the measurement noise. A number of case studies are carried out to demonstrate the effectiveness of the formulated deterministic and statistical damage identification algorithms.
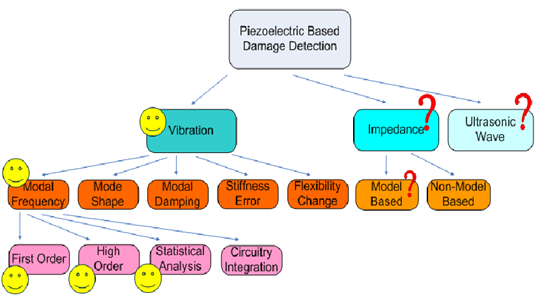