Smart Water Grid Anomaly Detection and Localization
With the advancement of Internet of Things (IoT) technology, a large amount of data, often referred to as Big Data, is collected and will need to be analyzed for improving system operations and water service to Singaporeans. This project is proposed to develop and apply data analytics to truly enable Smart Water Grid (SWG) as an integral component of smart Singapore. The objective of this project is to operationalize the outcomes of the ongoing collaboration and enhance the SWG operation. The specific objectives are (1) to enable the near real-time analysis of PUB SWG monitoring data; (2) to detect the anomaly events for the SWG operation; (3) to localize anomaly event hotspots for efficiently pinpointing the event in the field, and (4) to enhance PUB SWG operation and management with the hydraulic models calibrated using monitoring data.
This project has been jointly funded by Bentley Systems and Singapore National Research Foundation under its Competitive Research Programme (CRP) (Water) and administered by PUB (PUB-1804-0087), Singapore’s national water agency. We officially kicked off the project on March 1, 2020, since then, a team of R&D engineers have been hired and onboarded for developing the software solution that is developed and deployed to Singapore Government Commercial Cloud and tested/benchmarked in three water supply zones in Singapore.
In addition to software development, the team has actively pursued the through leadership by publishing peer-reviewed technical papers, giving presentations at international conferences and filing invention patents, as follows.
Peer-reviewed Journal Publication
- Wu, Z. Y. and He, Y. (2021). “Time Series Data Decomposition-based Anomaly Detection and Evaluation Framework for Smart Water System Management” ASCE J. Water Resources Planning and Management. DOI: 10.1061/(ASCE)WR.1943-5452.0001433
- Wu Z.Y., Chew A., Meng X., Cai J., Pok J., Kalfarisi R., Cheong L.K., Hew S.F. and Wong J.J. (2022)“Data-Driven and Model-Based Framework for Smart Water Grid Anomaly Detection and Localization” IWA AQUA -- Water Infrastructure, Ecosystems and Society Vol 71 No 1, 31 doi: 10.2166/aqua.2021.091.
- Chew A., Wu, Z. Y., Walski, T., Meng, X., Cai J., Pok, J. and Kalfarisi, R. (2022). “Daly model calibration with water loss estimation and localization using continuous monitoring data for water distribution networks” ASCE J. of Water Resources Planning and Management. doi: 10.1061/(ASCE)WR.1943-5452.0001546
- Meng X., Wu Z. Y., Cai J., Pok J., Chew A. and Kalfarisi R. (2022) "Improving Near Real-Time Anomaly Event Classification with Trend Change Detection for Smart Water Grid Operation Management" Urban Water Journal. DOI: 10.1080/1573062X.2022.2058565
- Kalfarisi R. Wu Z. Y., Cai J., Meng X. Pok J. and Chew A., (2022) “Predictive Modeling Framework Accelerated by GPU Computing for Smart Water Grid Data-Driven Analysis in Near Real-Time.” Advances in Engineering Software. vol. 173, pp. 103287 doi.org/10.1016/j.advengsoft.2022.103287.
- Chew A., Wu, Z. Y., Kalfarisi, R., Meng, X., Cai J., and Pok, J.(2023). “Acoustic Feature-based Leakage Event Detection in Near Real-Time for Large-Scale Water Distribution Networks”, IWA Journal of Hydroinformatics, https://doi.org/10.2166/hydro.2023.192
- Wu Z. Y., Chew A., Xue M., Cai J. Pok J., Kalfarisi R., Lai K.C., Hew; S. F. Wong J.J. (2023). "High Fidelity Digital Twin for Smart Water Grid Anomaly Detection and Localization". Sustainable Cities and Society. https://doi.org/10.1016/j.scs.2023.104446
- Chew A., Wu Z. Y., Xue M., Cai J. Pok J., Kalfarisi R. (2023). "Pressure-Based Demand Aggregation and Calibration of Normal and Abnormal Diurnal Patterns in Water Distribution Networks" ASCE J. Water Resources Planning and Management. https://doi.org/10.1061/JWRMD5.WRENG-5916
- Chew A., Wu Z. Y., Xue M., Cai J. Pok J., Kalfarisi R.(2023) "Acoustic Data Analysis Framework for Leakage Detection and Localization in Field Operational Water Distribution Networks", ASCE J. Water Resources Planning and Management. https://doi.org/10.1061/JWRMD5.WRENG-6122
- Zhang, A. H., Cao, F., Chew, A., Wu, Z. Y., Kalfarisi, R., Meng, X., Pok, J., Wong, J. M., Yang, C. (2024) "Near Real-Time Anomaly Event Localization by Pressure Drop Interpolation-Clustering and Parallel Optimization of Hydraulic Model Calibration" ASCE J. Water Resources Planning and Management. https://doi.org/10.1061/JWRMD5.WRENG-6464
Conference Presentations (33)
- 2 talks presented at Singapore International Water Weeks (SIWW) 2021
- 6 presentations given at Applied Research Seminar 2021
- 2 talks presented at ASCE EWRI 2021
- 1 presentation given at Singapore International Water Weeks 2022
- 2 talks given at ASCE EWRI 2022
- 6 talks given at WDSA-CCWI 2022 joint conference.
- 5 talks given at ASCE EWRI 2023 jointly with PUB officers.
- 1 talk given at AWWA ACE 2023
- 4 talks to be given at Singapore International Water Week (SIWW) 2024
- 3 talks to be given at WDSA-CCWI 2024
- 1 talk to be given at IWA WWCE 2024.
US Patents
- Evolutionary Deep Learning with Extended Kalman Filter for Effective Prediction Modeling and Data Assimilation. Awarded on Oct 10, 2023, by USPTO with Patent No.11783194 (uspto.gov)
- Anomaly Detection and Evaluation for Smart Water System Management, Awarded on April 16, 2024 by USPTO with Patent No. 11960254. ((https://ppubs.uspto.gov/dirsearch-public/print/downloadPdf/11960254)
- Systems, Methods, and Media for Near Real-Time Anomaly Event Detection and Classification with Trend Change Detection for Smart Water Grid Operation Management. Pending as US Patent Application No. 18/098,419.
- Systems, Methods, and Media for Calibrating a Model of a Water Distribution Network Utilizing Water Loss Estimation and Localizing Anomalies Using Continuous Monitoring Data. Pending as US Patent Application No. 18/098,400
- Near Real-Time Anomaly Event Localization by Pressure Drop Interpolation-Clustering and Parallel Optimization of Hydraulic Model Calibration. C-M Docket No. 107051-0645
- Generalized Acoustic Data Analysis Framework for Leakage Detection and Localization in Field Operational Water Distribution Networks. C-M Docket No. 107051-0647 .
Media Coverage
- Smart City and Infrastructure Evolution | Bentley Systems | Infrastructure Engineering Software Company
- Bentley Systems: 2023 is the start of infrastructure intelligence – FutureIoT
- Bentley Systems recognises YII 2023 as groundbreaking for infrastructure intelligence in Singapore | Water & Wastewater Asia (waterwastewaterasia.com)
- Bentley fills engineering resource gaps with infrastructure smarts | Control Global
- 2023 EWRI Congress (eventscribe.net)
Hydrant Selection Optimization for Flow Testing
We see hydrants on the side of streets. They do not look great, some of them are even rusty. However, it is hydrants that enable fire fighters to connect fire engine hoses with underground water pipelines and help with extinguishing the fire whenever it occurs. Fire hydrants are installed throughout urban water systems. It is standard practice to install hydrants every 500 ft in USA. Thus, there are hundreds and even thousands of hydrants installed in a urban water distribution system depending on the size of the city. Hydrant flow testing is not only routinely required (and widely adopted) for estimating the available fire flow, but also used for collecting pressure data for hydraulic model calibration, as well as flushing the pipelines to ensure adequate water quality. In order to collect good quality of field data, it is usually recommended that flow testing be conducted with the hydrants located at the outskirt of the distribution systems, and hydrant flow should result in a significant pressure drop, e.g. at least 10 psi or 70 kPa. With hundreds or even thousands of hydrants in a water distribution system, it is not straightforward task to select which hydrants are to be tested or flushed so that good quality of pressure data can be collected, and pipelines are cleaned up. In order to maximize the performance of the flow testing, in particular for the purpose of hydraulic model calibration, a new method is developed to search for a combination of the hydrants for flow testing. The implemented method is tested on a benchmark of water distribution system model. The results obtained for a benchmark system are compared with the hydrant selection solution by the experienced engineers. The performance of the optimized hydrant flow testing is significantly improved when comparing with the solution provided by the engineers.
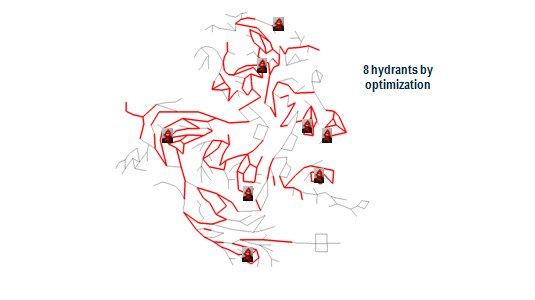
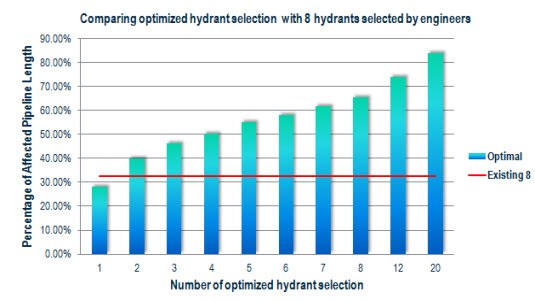
Software Prototypes:
- Darwin Sampler
- Software Tool Demo
- User Manual (draft)
Published Papers:
- Wu, Z. Y. and Song, Y. (2013) “Optimizing Selection of Fire Hydrants for Flow Tests in Water Distribution Systems.” The 12th International Conference on Computing and Control for the Water Industry, Sept. 7-9, 2013, Perugia, Italy.
Parallelized Hydraulic and Water Quality Analysis
Hydraulic and water quality models have been developed and used over decades for water distribution system (WDS) analysis. The models prove to be powerful tools for engineers to gain systematic understanding of the WDS conditions and conduct technically-sound WDS management. In particular, water quality modeling has become an increasing practice for water utilities. The model is not only a promising alternative for predicting disinfectant residuals in a cost-effective manner, but also a means of providing enormous engineering understanding in the dynamics of water quality variation and the sophisticated process of constituent reactions that occur in water distribution systems. With the models built with all-pipe in a system, it is very common that several minutes or even tens of minutes are taken for completing one water quality analysis run of a large model. The long computation time has been the bottleneck for water quality analysis to be efficiently executed as many scenarios as desired and/or integrated with iterative procedure for many optimization applications. In the meantime, computing hardware industry has offered desktops, laptops, tablets (or even smart phones) with dual-core or many-core computing power. This project developed the parallelization scheme for hydraulic and water quality simulations. It leverages the usage of the hardware computing capacity and provides more efficient analysis tools for engineers.
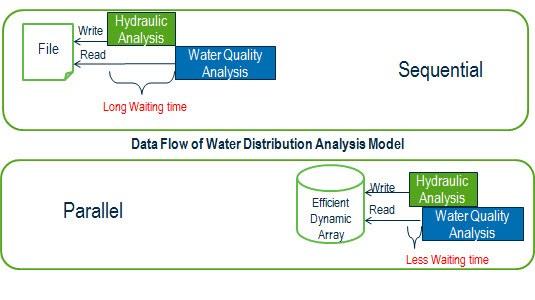
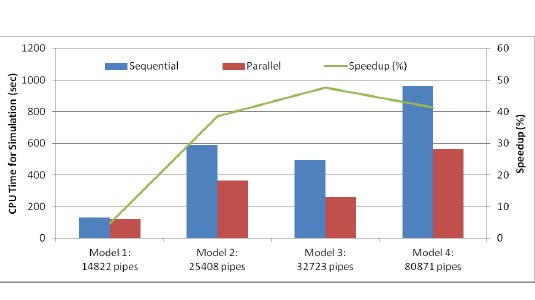
Published Papers:
-
Wu, Z. Y. and Elsayed, M. (2013) “Parallelized Hydraulic and Water Quality Simulation for Water Distribution Systems.” ASCE World Environmental and Water Resources Congress, May 20-23, 2013, Cincinnati, OH, USA
Pressure Logger Placement for Smart Water Systems
Smart water system has become a buzzing but ill-defined term. In many cases, smart water management is simply interpreted as automatic water metering and data-driven analysis, which is misleading and incomplete. The essence of smart water system management is to improve planning, scheduling and strategic decision-making for cost-effective and energy-efficient management of water systems. Water companies around the world are under increasing pressure to improve customer service. In order to achieve this there is a drive to increase network monitoring and analysis capabilities to proactively manage distribution systems and prevent failures in customer service occurring, thereby improving customer satisfaction levels. There is no doubt that being smart or intelligent at managing water systems requires good metering, monitoring technology and data analysis, but determining what kind of data and where to collect the data are among the foremost important issues to be addressed to be truly smart. Apart from water usage metering, pressure monitoring throughout a distribution network is critical to realize the smart water management.
A two-phase method is developed to determine the pressure logger placement. During the first phase, a database is generated by recording the pressure changes of each node for simulating randomly-generated leakage events, each of which can be represented as pressure dependent demand at one junction or multiple junctions. The database is persisted for the computation in the second phase, during which the optimization of the pressure logger locations is undertaken for the given number of pressure loggers, so that the optimized logger locations are able to cover the maximum number of leakage events. With the saved database, engineers can perform multiple optimization runs to determine the near-optimal locations of different numbers of pressure loggers for a system.
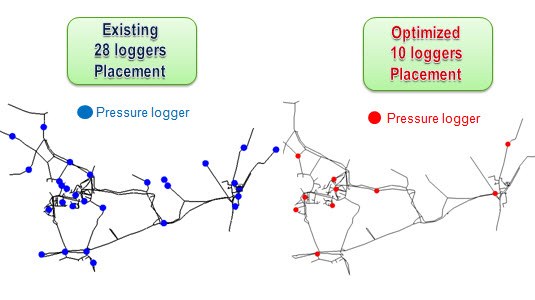
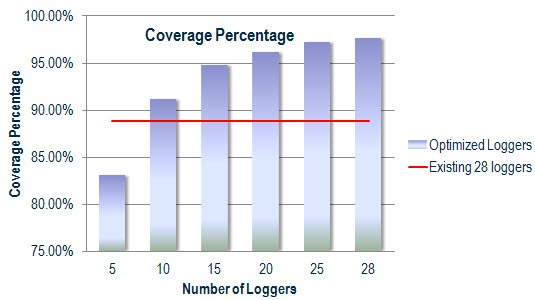
Published Papers:
-
Wu, Z. Y. and Song, Y. (2013) “Optimizing Selection of Fire Hydrants for Flow Tests in Water Distribution Systems.” The 12th International Conference on Computing and Control for the Water Industry, Sept. 7-9, 2013, Perugia, Italy.
Unknown Valve Status and Identification
Valves play an important role in water distribution system. There are many different types of valves that are installed in a water distribution system in order to deliver water supply to local communities. Valves, such as throttle control valve (TCV), are commonly used to regulate or control the flow of water, e.g. shutting off or redirecting the flow. They are the control points of a distribution system, and have become one of the most critical concerns for utilities dealing with sustainability, water main breaks, repairing and replacement activities. Knowing and tracking the exact valve locations and statuses bring many benefits for the improved management and operation of the distribution systems, including but not limited to the customer-call responsiveness, planned and unplanned maintenance, regulatory and non-regulatory compliance performance, more efficiently reducing total O&M costs, expedited with more accurate inventory and condition data and enhanced system integrity. However, it is not uncommon in a real system that the status and settings are unknown for some valves due to incomplete information for water asset management, missing records of maintenance work or system operation. With hundreds of valves installed throughout a system, only a limited number (just a handful) of valves are having unknown status or setting. The challenge is to identify, among hundreds of them, which valves are having the incorrect settings and what the correct status or settings are, so that they can be correctly represented in a model.
In this project, a new optimization model is developed to search for the unknown valves and the corresponding statuses/settings. The method is formulated within the model calibration framework of Darwin Calibrator. The same objective functions and the competent genetic algorithm as for the model calibration are employed for unknown valve identification. It is solved by optimizing the locations and settings of the unknown valves such that the difference between the observed and simulated values (flows and pressures) is minimized. Two case studies of real water systems are carried out to test the developed valve identification method.
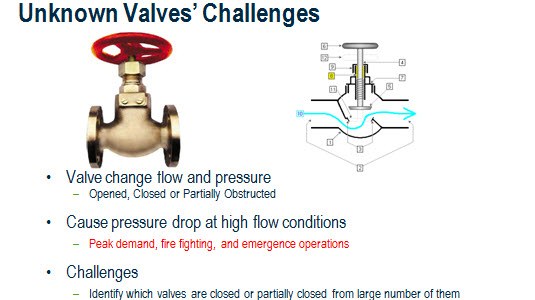
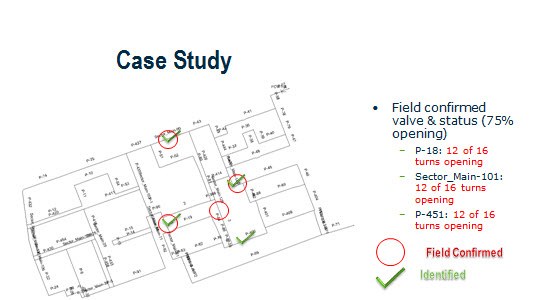
Published Papers:
-
Wu, Z. Y. and Song, Y. (2012) "Optimization Model for Identifying Unknown Valve Statuses and Settings", WDSA2012, Adelaide, Australia.
Pump Scheduling with GPU-based ANN
Pump scheduling (PS) is imperative for water utilities to achieve cost-effective and energy-efficient operation. Developing effective PS methods, especially for large water systems, has been a challenging problem in water distribution system analysis. Pump scheduling is to determine which pump is on or off at each of control intervals (typically every hour) over a control horizon (24 hours or longer), so that pumping energy cost and carbon footprint are minimized while satisfying the operational constraints. Real-time or online optimization is essential for realizing the benefits of pump scheduling for water distribution systems. Built on the previous research in GPU-based ANN modelling, A trained ANN meta-model is employed as the surrogate solver for evaluating the hydraulic responses of pump scheduling solutions. Darwin optimization framework is integrated to search for near-optimal pump operation schedule. Instead of taking several minutes to evaluate one PS solution by hydraulic model, using the trained ANN model needs only a fraction of second to evaluate one PS solution. Therefore it is possible to generate and evaluate tens of thousands of possible PS solutions in real time and minimize the energy cost and carbon footprint of large water systems.
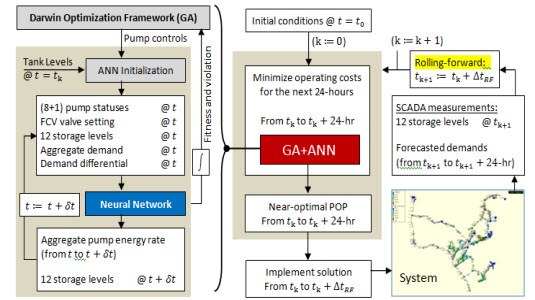
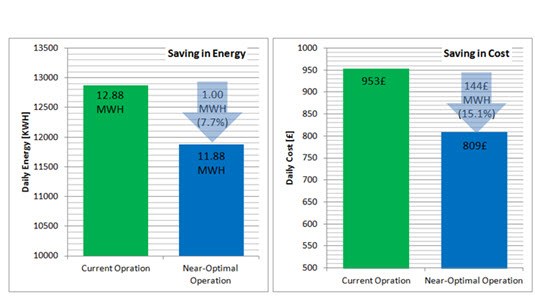
Published Papers:
- Wu, Z. Y. and Behandish, M. (2013) “Generalized GPU-Based Artificial Neural Network Surrogate Model for Extended Period of Hydraulic Simulation.” ASCE EWRI, May 20-23, 2013, Cincinnati, OH, USA.
- Behandish, M. and Wu, Z. Y. (2013) “Concurrent Pump Scheduling and Storage Level Optimization Using Meta-Models and Evolutionary Algorithm.” CCWI, Sept. 7-9, 2013, Perugia, Italy.
- Behandish, M. and Wu, Z.Y. “GPU-based Artificial Neural Network Configuration and Training for Water Distribution System Analysis ”, ASCE EWRI, May 21 – 24, 2012, Albuquerque, New Mexico, USA
- Wu, Z. Y and Behandish M. (2012) "Real-Time Pump Scheduling using Genetic Algorithm and Artificial Neural Network Based on Graphics Processing Unit", WDSA2012, Adelaide, Australia
- Wu, Z. Y and Eftekharian A.A. (2011). "Parallel Artificial Neural Network Using CUDA-enabled GPU for Extracting Hydraulic Domain Knowledge of Large Water Distribution Systems", EWRI, May 22-26, 2011, Palm Springs, California, USA.
Battle of Water Network II
The BWN-II calls for teams/individuals from academia, consulting firms, and utilities to propose a design methodology and apply it to a real water distribution system. The results of the BWN-II will be presented at a special session of the upcoming 14th Water Distribution Systems Analysis Symposium. The problem is to challenge the researchers to come up with solution to optimize (1) new pipe sizes; (2) parallel pipes; (3) pump station expansion; (4) tank expansion and (5) pump and valve controls over 168 hours (one week). The solution needs to satisfy the normal operating condition and abnormal conditions (power failure at any hour over 168 hours). Three criteria/objective functions, including least cost (capital and operational), least CO2 emission and least water age (retention time). I started to research the method to solve the problem.
Software Prototypes:
Published Papers:
-
Wu, Z. Y, Elsayed, M. and Song Y. (2012) "HPC Optimization for Solving BWN-II Problem", WDSA 2012, Adelaide, Australia
Leakage Detection Using Night Fire Flow Test
The WaterGEMS Darwin optimization pilot study for leakage hotspot detection within a United Utilities’ District Meter Area (DMA 02819) was passed to Bentley Systems in October 2011, eighteen months after Bentley Systems let a contract to WITSConsult Ltd to complete a study within 20 working days. There were several reasons for the delay arising from data errors in modeling and field test data supplied by the owner-operator. Over the contract period, the problems were resolved and/or worked around leading to the detection of several leaks that have subsequently been repaired in the field. All lessons learnt were passed to the UU owner operator with approval from Bentley Systems.
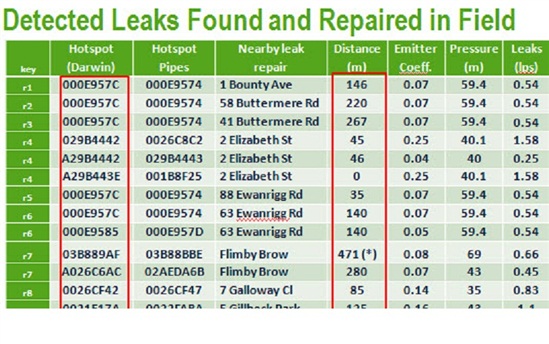
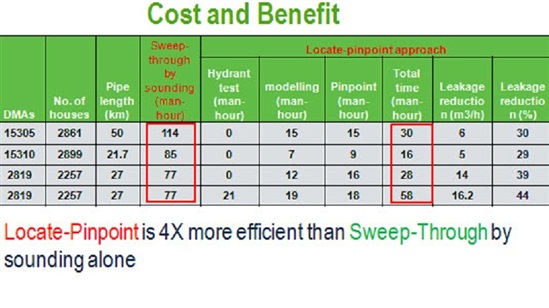
Published Papers:
- Wu, Z. Y. (2012) "Roles of Advanced Information Technology in Water Loss Management."IWA Water Loss 2012, Manila, Philippines.
- Wu, Z. Y., Sage, P. and Croxton, N. (2011). "Identifying Leakage Hotspots and Valve Status Using Night Fire Flow Testing Data" presented at 2011 World Environmental & Water Resources Congress on May 22-26, 2011, Palm Springs, California, USA
- Sage, N Croxton & Z Y Wu, "Recent developments in Leak Hotspot Detection using Network Model Optimization" CCWI 2011, Exeter, UK
- Sage, P, Wu, Z. Y. and Croxton, N. (2010) “Improves Leakage Detection and Model Calibration Using Fast Field Testing” EWRI Conference 2010, Providence, RI.